(Speaker 2018) Dr. Thomas Debray
Dr. Thomas Debray
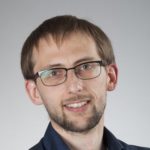
His background
Thomas Debray is an assistant professor experienced in statistical methods for prediction modeling research using large, clustered, datasets from e-health records or individual participant data meta-analysis. His higher education started with a Bachelor’s in Applied Computer Science at University College of Ghent, where he specialized in computer networks. He continued with an Master’s in Artificial Intelligence at Maastricht University, and a postgraduate Master’s in Clinical Epidemiology at Utrecht University. During his PhD at the Julius Center for Health Sciences and Primary Care, University Medical Center Utrecht, he started working on statistical methods for applying evidence synthesis in risk prediction, and developed several frameworks for evaluating and improving the performance of clinical prediction models.
The past few years, Thomas got increasingly involved in large international, projects and organizations where he advises health care professionals, researchers and decision makers in the analysis and interpretation of combined sources of data. Thomas is currently appointed at the Julius Center for Health Sciences and Primary Care, Oxford University, University College London and Cochrane Netherlands, where he investigates statistical methods for dealing with between-study heterogeneity, missing data, confounding and differential treatment response.
Website: https://www.thomasdebray.be
His talk
Clinical prediction models (CPM) are an important tool in contemporary medical decision making and abundant in the medical literature. These models estimate the probability/risk that a certain condition is present or will occur in the future by combining multiple patient characteristics, e.g. from medical history, physical examination or imaging tests. Unfortunately, many published CPM perform relatively poor in clinical practice, often due to the use of inappropriate statistical methodology and low quality data. In this talk, Thomas will illustrate how they can improve the validity and usefulness of developed prediction models by forming multidisciplinary and international collaborations to share expertise and patient-level data.